Computer algorithms that can spot patterns in vast amounts of data will drive the future of autism research and help scientists to understand autism’s true complexity and diversity, say AIMS-2-TRIALS researchers in a recent review article.
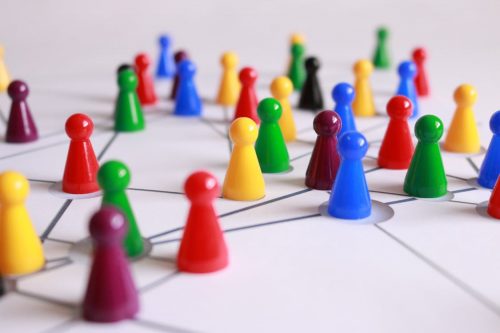
New approaches aim to identify traits common to sub-groups of autistic people
The algorithms’ power comes from their ability to integrate and analyse data on many different aspects of autism, all at once. The software can learn from the data and identify new patterns, which scientists will then use to group together autistic people who share similar traits and characteristics, in a process known as “stratification”. These groups will allow researchers to focus in on the biology that underpins each trait.
“There is not one autism, but many different autisms. To understand this, it is no longer enough to rely on human descriptions and intuition. We need to move beyond theories and be driven by the data. We need to let algorithms lead the way,” says Thomas Wolfers, a postdoctoral researcher at Radboud University, and first author of the review.
If the algorithm-based research successfully identifies sub-groups, it could inform the development of new treatments and supports that are tailored to each group’s needs. Such treatments could benefit people who want help with aspects of their autism, or co-occurring conditions, which are distressing or disabling. However, Wolfers cautions that it will be some time before any new treatments would be ready for use. In the meantime, findings from the research may allow autism to be diagnosed earlier than it is today, which could help families to understand and support their young children.
In the review, Wolfers and colleagues outline that most previous studies have used single measures of how autistic people think or behave, and which were often chosen to tie in with simple theories about the condition. These studies have typically compared the average value across one group, recorded from a given task or measure during an experiment, to the average of another. Although these group-based studies have helped to better understand who is likely to develop autism, they fail to capture the many differences between the people within each group and across the autism spectrum. According to the review, future research should collect and analyse much more data on each individual, with the goal of stratifying people into sub-groups based on their traits and biology. This is where algorithms come in.
Machine learning algorithms will be necessary to compute the larger amounts of data in future studies. The best results will come when studies not only involve many more participants, but also follow each person for a longer time than in previous research. Only by doing this can scientists begin to understand how autistic traits develop and change over time, says Wolfers.
The largest study in Europe to track development in autistic children and adults is known as LEAP (Longitudinal European Autism Project), and is part of AIMS-2-TRIALS. The many different measurements that it collects will give a fuller and deeper picture of autistic traits and characteristics, in a method known as “deep phenotyping”. All of the data will be stored securely and anonymised, so that it is not possible to identify the people who took part. The ultimate goal is to identify biological markers that could be used to predict if a person is likely to develop co-occurring symptoms or to benefit from a specific treatment.
The review paper is “in press” in the journal Neuroscience and Biobehavioural Reviews.